Media Mix Modelling (what, how & why)
Media Mix Modeling, Marketing Mix Modeling, or MMM, is a privacy-friendly and statistic driven way to measure how marketing and advertising campaigns perform. Product, price, place and promotion are all analyzed by MMM to help achieve a goal.
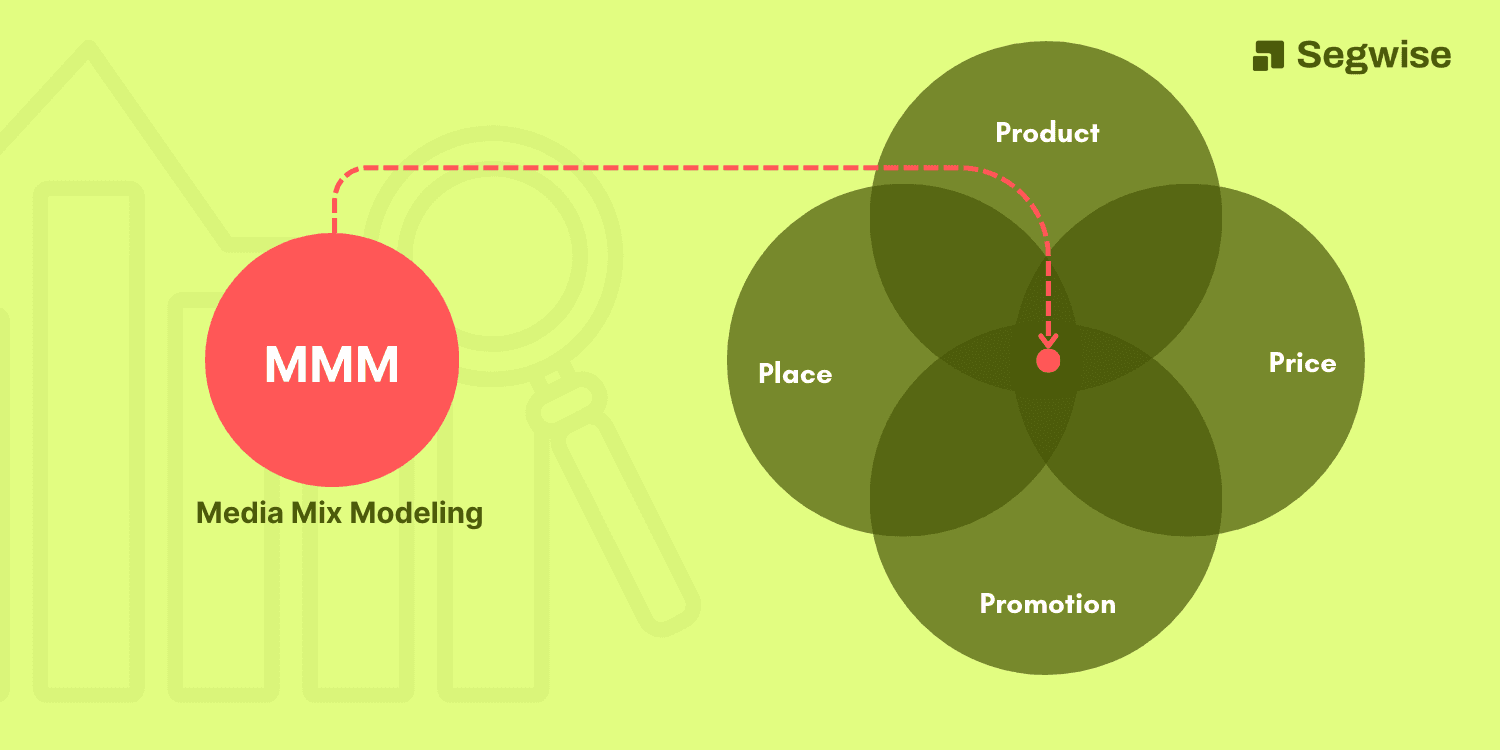
For instance, it can explain how pricing change, customer demographics, advertising expenditures, and other factors influence your sales. Additionally, it can predict potential outcomes if there are any changes.
Marketing Mix Modeling (MMM) is a useful tool in marketing analytics that helps measure the return on investment (ROI) for advertising. It shows how different marketing strategies affect results. Knowing how this model works is important for businesses that want to increase profits and improve their marketing efforts.
Also read Future of Game Marketing: Next Gen MMM
How MMM Works
Marketing Mix Modeling (MMM) uses statistical analysis, mainly multilinear regression, to examine data from marketing campaigns. This helps businesses understand the link between spending on different marketing channels and results, like revenue or user acquisition.
By studying these links, companies can make better decisions to improve their marketing strategies and get more value. MMM also helps determine which marketing efforts work best, so businesses can allocate their budget to boost performance and growth.
MMM Advantages
- Measuring both online and offline marketing channels.
- Shows how different variables relate to each other
- Tracks both online and offline conversions
- Estimates media saturation and effectiveness, helping marketers identify the best investment levels
- Advanced methods offer scenario planning and budget optimization, allowing for simulations to predict business outcomes
- Considers factors that directly affect ROI
- Protects user privacy by not using personal information
MMM Disadvantages
- Needs a lot of historical data
- Depends on several assumptions for factors outside marketing
- Offers reports infrequently
- Doesn't analyze how channels interact
- Lacks insights into brand or messaging effectiveness
- Ignores customer experience considerations
MMM Framework
MMM framework includes the marketing channels you use, the ad spend for each channel, and past campaign results. The goal is to help the marketing team figure out what factors will affect how successful future campaigns are.
The MMM data often used in marketing mix modeling includes:
Mobile Attribution Numbers: This is data you gather yourself or data from platforms like Facebook, Google Ads, TikTok, or any other self-attributing network you work with, or from the SDKs of your mobile measurement partner, showing your digital advertising results.
Seasonality: Many mobile apps are affected by seasonality. From holiday trends to events like back to school, including seasonal data in your MMM feed can help predict how these events may influence your marketing efforts.
Press: The impact of press coverage varies by industry, but if your team is creating quality bylines, press releases, and guest posts, it's worth measuring. We also suggest checking your app’s daily active users to see if and when press coverage affects app engagement.
Phases of MMM Implementation
Implementing MMM, requires a methodical approach to ensure optimal results and insights. This involves several critical steps:
- Clearly define the objectives: Start by setting specific goals and objectives for what you want to achieve with MMM. Understanding the key questions you aim to answer will guide the modeling process effectively.
- Data collection and preparation: Compile a comprehensive dataset that includes all relevant data sources. This involves gathering historical data on sales, marketing activities, and external factors. Ensure the data is clean, consistent, and covers a significant time period to facilitate accurate analysis.
- Choose the right modelling technique: Select an appropriate statistical method that fits your data and objectives. Common techniques include regression analysis and machine learning models, each offering different strengths based on the complexity and nature of the data.
- Model building and calibration: Develop the MMM by incorporating the chosen methodology. Repeatedly test and refine the model to ensure it accurately represents the relationships between variables and generates reliable predictions.
- Insights generation and application: Analyze the output to derive actionable insights. Utilize the model to identify which marketing channels are most effective, optimize the allocation of marketing budgets, and simulate the impact of different strategies.
- Continuous evaluation and adjustment: Regularly assess the model’s performance and adjust it as necessary. As market conditions and business objectives change, the model must evolve to remain accurate and relevant.
By following these steps, organizations can effectively leverage MMM to drive better decision-making and improve marketing ROI.
MMM vs MTA
MMM helps businesses understand the impact and ROI of different marketing channels, showing which ones boost sales and engage customers the most.
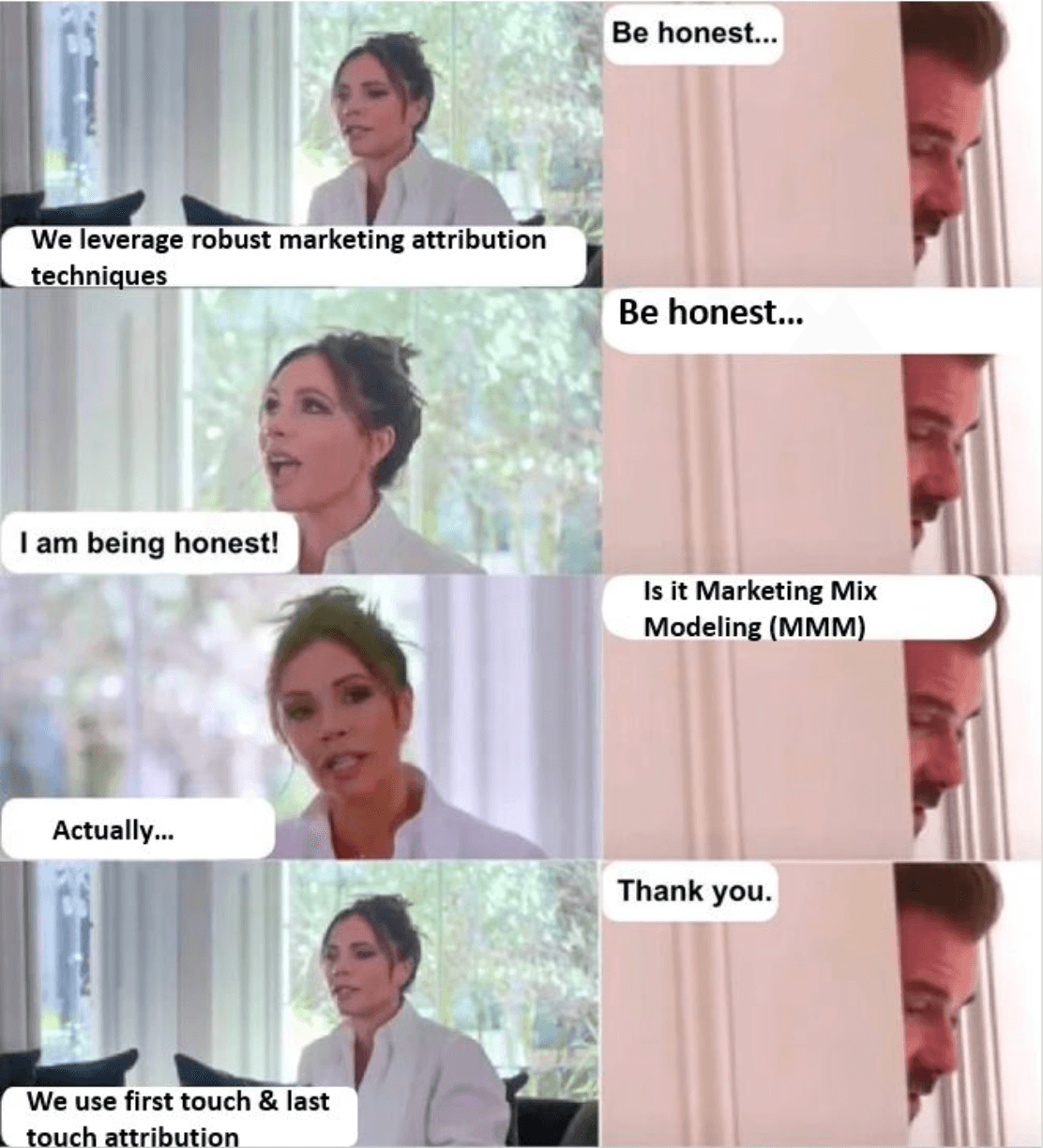
However, MMM requires a lot of data to make accurate models and depends on historical assumptions, which might not always predict the future well. When comparing MMM and MTA (Multi-Touch Attribution), MMM focuses on the broader, long-term effects of marketing strategies, while MTA offers detailed insights into individual customer touchpoints.
MMM is great for spotting overall trends and campaign effectiveness, whereas MTA helps understand specific customer interactions leading to conversions. This distinction helps businesses choose the right model based on their goals and data availability.
Conclusion
MMM is not for everyone as it needs a lot of good-quality data. This can be a challenge if you have a small marketing budget or if your organization has just started media buying on a large scale, as most models need at least two years of historical data to make predictions.
If you have access to all the required data, MMM can work wonders. While it demands high-quality data, investing in data skills and improving organizational capabilities can be extremely valuable.
Frequently Asked Questions (FAQs)
What are the advantages of Media Mix Modeling?
MMM helps optimize marketing budgets, estimates ROI across channels, respects user privacy, and enables scenario planning for different marketing strategies.
What kind of data is needed for MMM?
MMM requires at least 2 years of historical data including sales, marketing spends across channels, seasonality, and external factors like pricing and promotions.
Is Media Mix Modeling suitable for small businesses?
MMM typically suits medium to large businesses with robust historical data. Smaller businesses may find it challenging due to limited data and resources.