"Without data, you’re just another person with an opinion.” — W. Edwards Deming.
This quote perfectly captures the reality of mobile gaming today. With user acquisition costs soaring and competition fiercer than ever, relying on guesswork is no longer an option.
Data-driven decisions, powered by predictive analytics, have become the standard for developers and marketers alike. From optimizing marketing strategies to boosting ROI and maintaining user engagement, predictive analytics holds the key to unlocking growth and sustainability for your gaming app.
In this blog, we'll explore how predictive analytics for marketing can transform your gaming app's acquisition strategies.
Let’s get started!
What is Predictive Analytics?
Predictive analytics involves using historical data and algorithms to forecast future outcomes. It’s crucial in gaming app marketing as it helps predict user behavior, campaign performance, and market trends. By transforming raw data into actionable insights, predictive analytics allows you to:
Anticipate user churn and adjust campaigns.
Predict ROAS for your campaigns.
Maximize ROI by optimizing marketing spend.
With predictive analytics, marketing becomes more precise, cost-effective, and aligned with user needs. Now that we’ve covered the basics of predictive analytics, let’s dive into the stages that build its foundation.
Stages of Data Analytics Maturity in Marketing
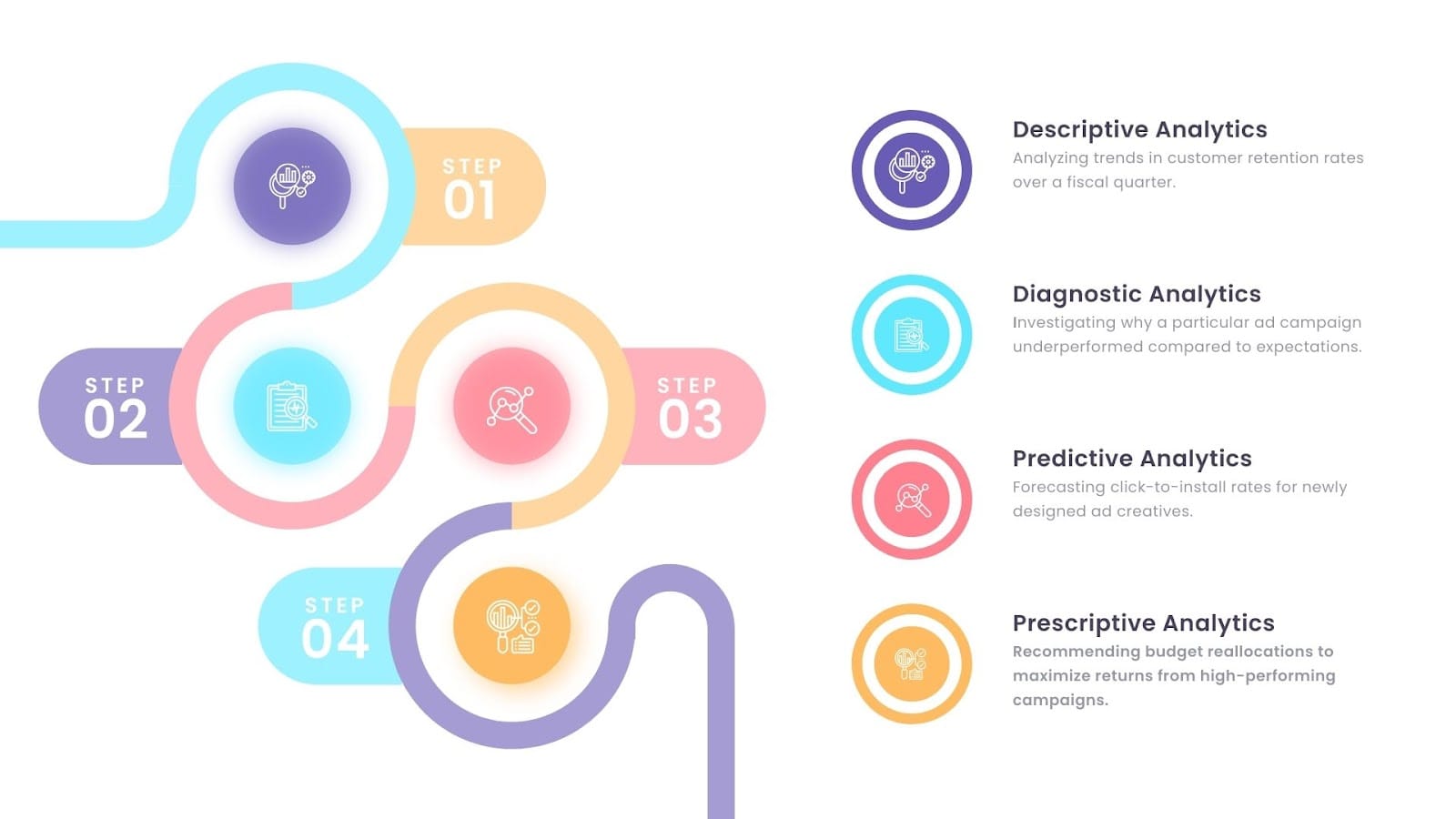
Understanding predictive analytics requires a clear grasp of its foundational stages, as depicted in Gartner's data analytics maturity graph. Each stage represents a step forward in transforming raw data into actionable insights, serving distinct purposes in marketing decision-making:
1. Descriptive Analytics (What happened?)
Descriptive analytics captures the current and past states of marketing campaigns, customer retention, attrition rates, or sales KPIs. It provides a factual summary of events, enabling teams to review historical performance.
Example: Analyzing trends in customer retention rates over a fiscal quarter.
2. Diagnostic Analytics (Why did it happen?)
Diagnostic analytics moves beyond the "what " and identifies the reasons behind specific outcomes. This stage equips teams with a deeper understanding of cause-and-effect relationships within their campaigns.
Example: Investigating why a particular ad campaign underperformed compared to expectations.
3. Predictive Analytics (What will happen?)
Predictive analytics leverages insights from the descriptive and diagnostic stages to forecast future outcomes. It empowers marketers to anticipate trends, make informed decisions, and optimize their strategies.
Example: Forecasting click-to-install rates for newly designed ad creatives and estimating the potential ROAS for each campaign.
4. Prescriptive Analytics (What should we do?)
Prescriptive analytics provides actionable recommendations by analyzing historical data alongside external information. Advanced tools and technologies guide decision-making, helping businesses mitigate risks or seize opportunities.
Example: Recommending budget reallocations to maximize returns from high-performing campaigns.
Each stage builds toward predictive analytics, empowering marketing teams with forward-looking insights. With these stages in mind, let’s discuss how you can prepare your data for predictive modeling.
Data Collection and Preparation for Predictive Modeling
Achieving accurate predictive modeling begins with collecting and preparing high-quality data. Here's how to ensure your data is ready for actionable insights:
Key Data Types to Collect:
User Interactions: Collect granular metrics such as clicks, app installs, in-app purchases, session frequency, daily active users (DAU), retention rates, time spent per session, and user progression through app levels.
Ad Performance: Gather insights on impressions, click-through rates (CTR), cost-per-install (CPI), cost-per-click (CPC), cost-per-acquisition (CPA), and return on ad spend (ROAS).
Spending Data: Track metrics like lifetime value (LTV), average revenue per user (ARPU), subscription revenue, and in-app spending trends.
Behavioral Trends: Analyze session duration, churn rates, drop-off points, purchase patterns, and user retention cohorts to identify engagement drivers.
Demographics and Device Data: Include user location, age, device type, operating system, and time of day usage patterns for contextual understanding.
Essential Tools and Techniques:
Leverage Mobile Measurement Partners (MMPs) like Adjust and AppsFlyer for precise tracking.
Utilize marketing analytics platforms like Segwise for streamlined data management and analysis.
Data Cleaning and Preprocessing Steps:
Eliminate duplicates and filter out irrelevant information.
Normalize datasets to maintain uniformity across variables.
Resolve missing values to minimize inaccuracies in the predictive model.
Refining your data with these methods ensures a robust foundation for building reliable and impactful predictive models. Once your data is ready, the next step is to understand why building predictive models is essential for marketing success.
Why Build Predictive Models in Marketing?
With Predictive models, you can identify high-value customers by analyzing past behaviors and trends. By predicting which customers are more likely to convert, marketers can allocate resources more efficiently and target the right audience with the right message. They help marketing teams achieve two key objectives:
User Acquisition Optimization
Understanding user behavior early on can be advantageous for your campaigns. By identifying high-potential users right from the start, you can focus your resources where they matter most—on the audiences likely to bring the greatest value.
At the same time, you can fine-tune bids, adjust creatives, and refine targeting for segments that aren’t performing as expected. This proactive approach not only helps you optimize costs but also ensures you’re reaching the right people with the right message, creating more meaningful connections while improving overall campaign efficiency. It’s about working smarter, not harder, to make every marketing dollar count.
Examples:
Optimizing Cross-Channel UA:
MMM insights show TikTok video ads capture 90% of ad recall and 80% of awareness within six seconds. Prioritize short-form, engaging creatives that resonate with high-intent users, ensuring every dollar works toward meaningful conversions.Allocating Budgets for Maximum ROI:
Identify saturation points with MMM cost curve analysis. For example, scale TikTok spend strategically when incremental returns are high, avoiding wasted ad spend and maximizing campaign efficiency.Refining Campaign Performance:
Use MMM to test and refine ad creatives, focusing on styles and formats proven to drive installs. By doubling down on what works, you ensure sustained acquisition of users who align with your long-term goals.
Intent-driven UA strategies built on actionable insights not only boost ROI but also deliver sustained growth by targeting the right users at the right time.
Privacy-Centric Advertising
With increasing privacy regulations like GDPR and CCPA, marketers need innovative approaches to targeting audiences without relying on personal identifiers. Predictive models, which focus on behavioral data, enable a privacy-centric advertising strategy that balances compliance with performance.
Key Actions:
Behavioral Clustering for Audience Segmentation:
Predictive analytics enables gaming app marketers to attract high-value players by analyzing behavior patterns instead of relying on personal data. Group players based on interactions like time spent in-game, level completion, or event participation to create precision-targeted campaigns.
Example: Identify users engaging with seasonal events but not making purchases. Target them with exclusive in-game offers tied to event participation, driving both acquisition and early-stage engagement.
Campaign Expansion Without Compromising Privacy:
Predictive analytics models identify behavioral patterns that mimic high-value users, enabling ad networks to target similar audiences effectively without sharing personal user data.
Example: Instead of sending personal identifiers to ad networks, anonymized behavioral clusters—such as frequent event participants or players with high in-app engagement—are used to find lookalike audiences for campaign expansion, driving conversions while safeguarding privacy.
Privacy Compliance with Performance Retention:
Behavioral data anonymization ensures compliance with privacy regulations like GDPR or CCPA. By focusing on aggregated patterns rather than individual identities, gaming campaigns can optimize retention strategies effectively while maintaining user trust.
This approach allows gaming marketers to scale campaigns and retain users while staying privacy-compliant and enhancing audience targeting precision.
To fully utilize predictive analytics, it’s crucial to identify the right metrics and KPIs to measure performance effectively.
What Should You Measure?
Effective predictive modeling requires identifying and tracking the right metrics and KPIs to evaluate performance accurately:
Metrics: Early Indicators
Click-to-Install (CTI):
Formula: Number of installs ÷ Number of ad clicks.
Purpose: Evaluate audience relevance and creative effectiveness.
Click-Through Rate (CTR):
Formula: Number of clicks ÷ Number of ad views.
Purpose: Measures ad creative engagement at the top of the funnel.
Cost Per Install (CPI):
Formula: Ad spend ÷ Total installs.
Purpose: Tracks acquisition costs for paid installs.
Retention Rate:
Formula: [(CE – CN) ÷ CS] × 100.
CE (Customers at End): Number of users at the end of a specific period.
CN (New Customers): New users acquired during that period.
CS (Customers at Start): Number of users at the beginning of the period.
Purpose: This metric measures how well your app retains users over time.
KPIs: Confident Predictors
KPIs provide a deeper, long-term understanding of marketing campaign success.
Tier 2 KPIs (Medium Confidence):
Cost per Key Actions: Tracks costs for specific user behaviors (e.g., tutorial completions or product views).
Cost per Retained User (Day X): Measures spending efficiency for users retained on specific days.
Purpose: Offers medium confidence for profitability benchmarks.
Tier 1 KPIs (High Confidence):
Return on Ad Spend (ROAS):
Formula: Revenue ÷ Marketing spend.
Purpose: Directly links marketing efforts to profitability.
Lifetime Value (LTV):
Formula: Average conversion value × Average conversions × Customer lifetime.
Purpose: Predicts long-term revenue and overall campaign value.
With the right metrics in place, it’s also vital to continually refine predictive models for ongoing success.
Continuous Improvement of Predictive Models
To maintain effectiveness, predictive models must be regularly updated:
Retrain with Fresh Data: Ensure models adapt to evolving user behaviors.
Leverage Acquisition Metrics: Use performance insights to refine targeting strategies.
Overcome Challenges: Address issues like data sparsity and shifts in market trends.
Beyond technical accuracy, predictive analytics opens doors to unparalleled personalization in marketing efforts.
Personalization of Marketing Efforts
Personalized acquisition strategies have become indispensable for brands seeking to connect with their audiences. Predictive analytics for marketing plays a crucial role in making these strategies effective, enabling businesses to anticipate customer behavior, refine campaigns, and deliver tailored experiences.
Let’s explore how predictive analytics empowers marketing personalization with examples from the mobile gaming industry:
Product Development
Predictive analytics helps mobile game developers identify emerging trends and design games that resonate with future audiences. For example, Supercell, creators of popular titles like Clash Royale, use analytics tools to track player feedback and gameplay patterns. By analyzing these trends, they can introduce game features and updates aligned with player preferences, keeping their games fresh and engaging.
Customer Segmentation
Segmentation powered by predictive analytics allows mobile gaming companies to classify players into meaningful categories. Zynga, known for games like Words With Friends, uses machine learning to segment its players into groups such as “daily players” and “occasional spenders.” This segmentation helps them design targeted campaigns, offering exclusive deals or rewards tailored to each group, leading to higher engagement and monetization.
Uplift Modeling
Forecasting campaign effectiveness is critical in the competitive gaming space. For example, King, the developer of Candy Crush Saga, uses uplift modeling to predict the impact of in-app promotions on player engagement. Optimizing these campaigns reduces the time spent on manual testing and ensures that offers are highly relevant, increasing in-app purchases and retention rates.
Recommendation Systems
Predictive analytics power recommendation engines, ensuring players find the right games and in-game items. Playrix, the creator of Gardenscapes, uses algorithms to recommend personalized game levels or booster packs based on players' past activities. These recommendations improve the player experience and drive sales of in-game purchases, fostering loyalty.
Lead Prioritization
For mobile game publishers, identifying high-value users early is key. A gaming platform like Game Insight uses predictive analytics to prioritize leads by identifying players most likely to spend on premium features. By targeting this audience with onboarding incentives and exclusive in-game offers, they improve conversions and nurture long-term engagement.
Churn Prediction
High churn rates are a challenge for the gaming industry. Predictive models help developers spot players at risk of leaving. For instance, Scopely, behind games like Marvel Strike Force, uses data to detect disengagement patterns. They proactively send tailored incentives—like free character upgrades or time-limited offers—to re-engage players and prevent churn, effectively extending the game’s lifecycle.
Ad Personalization
Personalized ad strategies can significantly boost acquisition efforts. Take Rovio Entertainment, the makers of Angry Birds. Using predictive analytics, they craft ad campaigns optimized for different demographics. By tailoring ad content to align with player preferences (e.g., showing gameplay clips of specific levels or characters), Rovio improves ad click-through rates and conversion efficiency.
Predictive analytics helps companies deeply understand players, anticipate needs and deliver targeted campaigns. It enhances engagement with tailored offers, reduces churn through timely actions, and enables developers to attract and retain players effectively.
Also Read: Personalization Techniques for In-App Purchases in Mobile Games
Future Trends in Predictive Analytics for Gaming Apps
Predictive analytics for marketing is revolutionizing user acquisition in gaming apps, offering data-driven strategies to attract and retain players. Key trends include:
Personalized Player Experiences: Tailored gameplay, dynamic difficulty adjustments, and personalized recommendations enhance engagement and retention, driving organic growth.
AI-Driven Precision: Advanced AI and machine learning refine targeting, predict lifetime value (LTV), and optimize ad spending for efficient acquisition.
Dynamic In-Game Advertising: Behavior-based ad placements boost conversions while preserving the gaming experience.
Cross-Channel Integration: Unified marketing across platforms ensures campaigns reach the right audience at the right time.
Churn Prediction: Identifying at-risk players enables proactive retention efforts, strengthening long-term engagement.
Privacy Compliance: Tools like differential privacy build trust and ensure data security, a key factor in user acquisition.
By leveraging these innovations, predictive analytics transforms acquisition strategies, unlocking cost-effective growth and new market opportunities.
Conclusion
Predictive analytics is reshaping marketing by transforming data into actionable insights. It enables smarter acquisition strategies, personalized campaigns, and proactive user engagement. For mobile gaming and beyond, it drives better ROI, reduces inefficiencies, and ensures compliance with privacy regulations, making data-driven decisions a necessity for sustainable growth.
Segwise accelerates this transformation. By integrating data from MMPs like Adjust and Appsflyer, Segwise empowers businesses with AI-driven insights, predictive ROAS analytics, and creative optimization tools.
Our proactive monitoring and seamless integrations ensure precise targeting, enhanced ad performance, and efficient budget allocation. For businesses aiming to optimize acquisition efforts and maximize returns, we offer the data-driven edge needed to succeed.
Start optimizing your acquisition efforts today with Segwise. Sign up now for a 14-day free trial and experience data-driven marketing at its best!